Transformers vs. Graph Neural Networks (GNNs): The AI Rivalry That’s Reshaping the Future
- Debopriya Lahiri
- Mar 20
- 4 min read
Updated: 2 days ago
In a futuristic AI lab, two legendary models are deep in discussion.
"You're powerful, but you don’t understand structure," says Graph Neural Network (GNN), adjusting its weighted edges.
"And you? You’re stuck in your graphs while I rule language, vision, and speech!" Transformer boasts, its self-attention layers glowing.
This is not just an ordinary conversation—this is a battle between two of the most powerful architectures in artificial intelligence today. One dominates text and vision, while the other unravels complex networks like never before. But which one truly holds the key to the future?
To understand, let’s rewind to where it all began.
The Rise of Transformers: "Attention Is All You Need"
The Year: 2017
The Place: Google Brain
A team of researchers, including Ashish Vaswani, Noam Shazeer, Niki Parmar, and others, released a groundbreaking paper titled "Attention Is All You Need." It introduced the Transformer architecture, which replaced traditional recurrent neural networks (RNNs) and long short-term memory networks (LSTMs) with a new, powerful approach: self-attention.
Until this point, AI models struggled to process long texts efficiently. But Transformers changed everything by enabling parallel processing rather than sequential step-by-step computation.
How Transformers Work: Breaking It Down
Self-Attention: Instead of reading one word at a time, Transformers analyze all words simultaneously, figuring out which words are important for a given task.
Positional Encoding: Since Transformers don’t process words in order like humans, they need extra information to know which words come first and how they relate to each other.
Multi-Head Attention: Instead of focusing on just one aspect, the model looks at multiple relationships at once. Think of it as reading a book while also scanning for key phrases.
Knowledge Box: The Power of Transformers
Models Built on Transformers: BERT, GPT-4, ViTs (Vision Transformers)
Applications: Chatbots, AI assistants, image recognition, content generation
Weaknesses: High computational cost, struggles with structured data
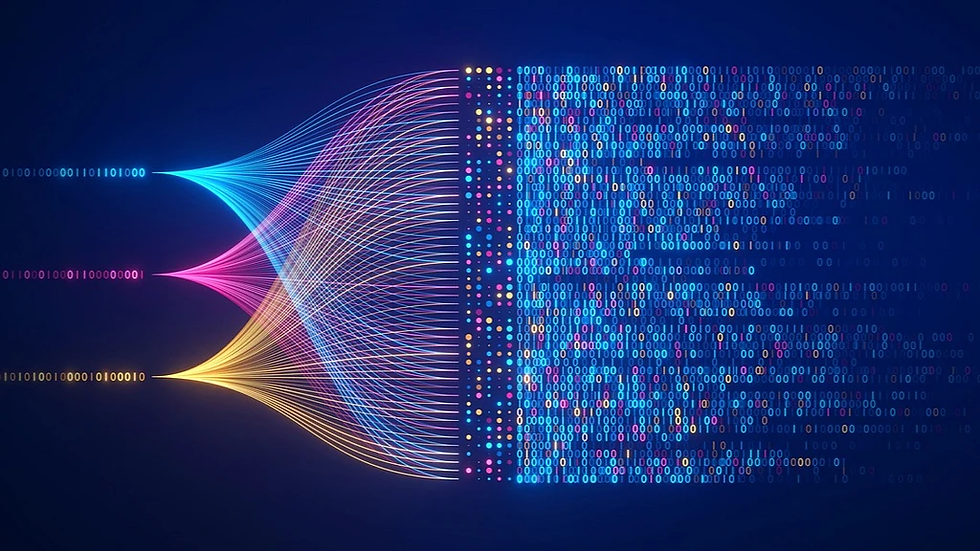
Enter GNNs: The Architect of Networks
The Year: 2005 - 2017
The Researchers: Scarselli et al. (2005), Thomas Kipf & Max Welling (2017)
While Transformers were revolutionizing text and vision, another innovation was quietly reshaping AI: Graph Neural Networks (GNNs).
Unlike traditional AI models that work on grids (like images) or sequences (like text), GNNs are built to analyze relationships between entities—perfect for social networks, molecular structures, and recommendation systems.
How GNNs Work: The Magic of Message Passing
Nodes and Edges: A graph consists of nodes (data points) and edges (connections). Imagine a social network where people (nodes) are connected through friendships (edges).
Message Passing: Each node collects and updates information from its neighbors, much like how people learn from their surroundings.
Graph Convolutions: Instead of scanning images, GNNs scan entire networks, capturing deeper patterns.
Knowledge Box: The Strength of GNNs
Popular Models: Graph Convolutional Networks (GCNs), Graph Attention Networks (GATs)
Applications: Social media analysis, drug discovery, fraud detection Weaknesses: Harder to parallelize, struggles with sequential data

Which AI Model Would You Choose?
If you were building a chatbot like ChatGPT, which model would you use?
A) Transformer
B) GNN
If you were analyzing friendship patterns on Facebook, which model would be more useful?
A) Transformer
B) GNN
(Scroll down for answers!)
Transformers vs. GNNs: The AI Showdown in Storytelling
Once upon a time, two powerful AI warriors—Transformers and Graph Neural Networks (GNNs)—set out on a mission to conquer the world of data!
Transformer: The Master of Language & Sequences
Meet Transformer, the storyteller of the AI kingdom! It reads books, translates languages, and even chats like a human.
Writes like Shakespeare: ChatGPT, BARD, and other AI writers owe their magic to it.
Understands speech: Powers Alexa, Siri, and voice assistants.
Deciphers proteins: Helps in drug discovery by reading biological sequences like a pro.
Creates videos & images: Fuels AI art, deepfakes, and video generation.
GNN: The Web Weaver of Relationships
GNN, on the other hand, is a master of connections, thriving in networks and graphs. It sees beyond individual data points and finds patterns in relationships.
Detects fraud: Spots suspicious transactions in banking networks.
Recommends friends: Powers Facebook, LinkedIn, and social networks.
Develops new drugs: Analyzes molecular structures to design life-saving medicines.
Predicts game strategies: Analyzes player movements in sports analytics.
Both AI warriors work together, transforming raw data into knowledge and predictions! While Transformers craft powerful stories and predictions, GNNs uncover hidden relationships—making them unstoppable in AI research!
A New AI Era: Can Transformers and GNNs Work Together?
Despite their differences, researchers are now combining Transformers and GNNs to create hybrid models capable of solving more complex problems.
For example: In drug discovery, a GNN can analyze molecular structures while a Transformer helps understand medical literature.
In recommendation systems, GNNs model relationships between users, while Transformers process customer reviews.
This neurosymbolic AI approach represents the future—where Transformers and GNNs work not as rivals but as partners.
Fun Fact Corner: AI in Unexpected Places
Did you know? NASA is experimenting with Graph Neural Networks for space exploration! GNNs are used to map and predict asteroid orbits, helping scientists plan safe spacecraft trajectories.
Transformers in Art? OpenAI’s DALL·E uses Transformer models to generate realistic AI-created paintings.
Cybersecurity Watch: Banks use GNNs to detect fraud by analyzing customer transaction networks, while Transformers help process suspicious emails and messages.
Final Ending: The Future Belongs to Both
Rather than choosing one over the other, AI professionals and students should master both Transformers and GNNs to unlock new possibilities. Whether you're working in NLP, healthcare, cybersecurity, or astrophysics, understanding these architectures will put you at the forefront of AI innovation.
Quiz Answers:
1. A) Transformer (Chatbots need sequence-based understanding.)
2. B) GNN (Social networks involve complex relationships.)
What’s Next?
AI is evolving every day. The next step? Hybrid models that merge the strengths of Transformers and GNNs.
What do you think? Would you use Transformers, GNNs, or a combination of both for your next AI project?
1
Searing the Beef
Sear beef fillets on high heat for 2 minutes per side to form a golden crust. Let it cool before proceeding to keep the beef tender.
1
Searing the Beef
Sear beef fillets on high heat for 2 minutes per side to form a golden crust. Let it cool before proceeding to keep the beef tender.
1
Searing the Beef
Sear beef fillets on high heat for 2 minutes per side to form a golden crust. Let it cool before proceeding to keep the beef tender.
1
Searing the Beef
Sear beef fillets on high heat for 2 minutes per side to form a golden crust. Let it cool before proceeding to keep the beef tender.
Notes
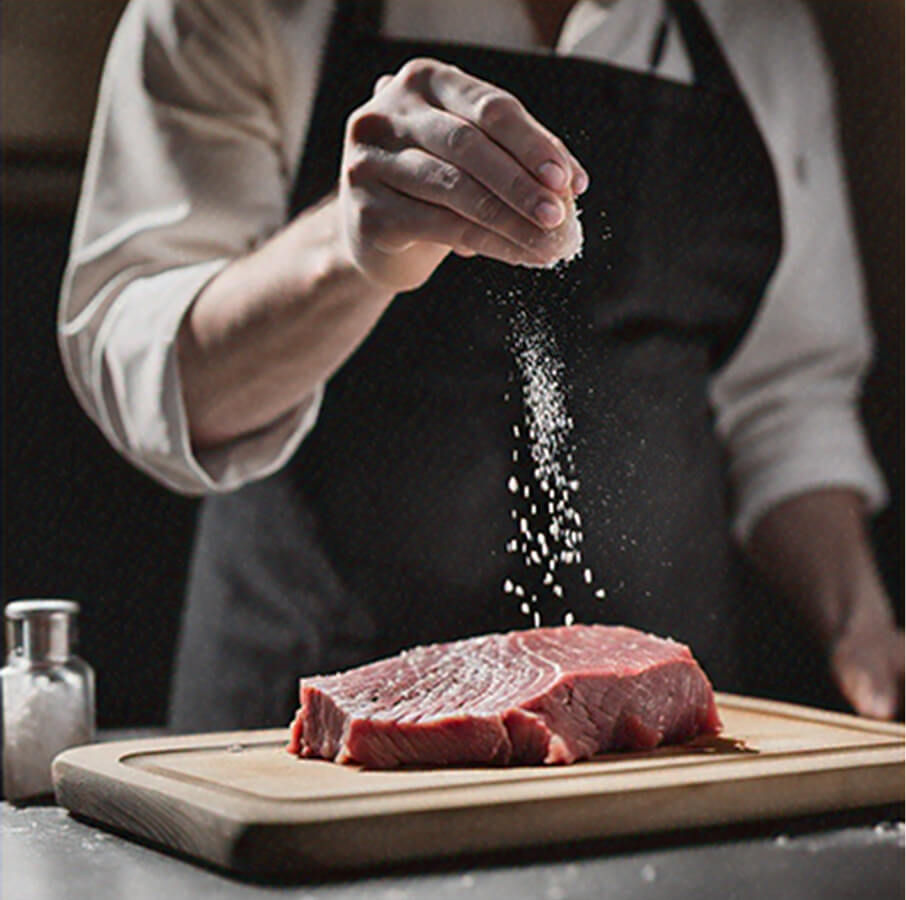


1
Season the good fresh beef fillets with salt and black pepper. Heat olive oil in a pan over high heat and sear the fillets for 2 minutes per side until it fully browned. Remove the beef from the pan and brush with a thin layer of mustard. Let it cool.
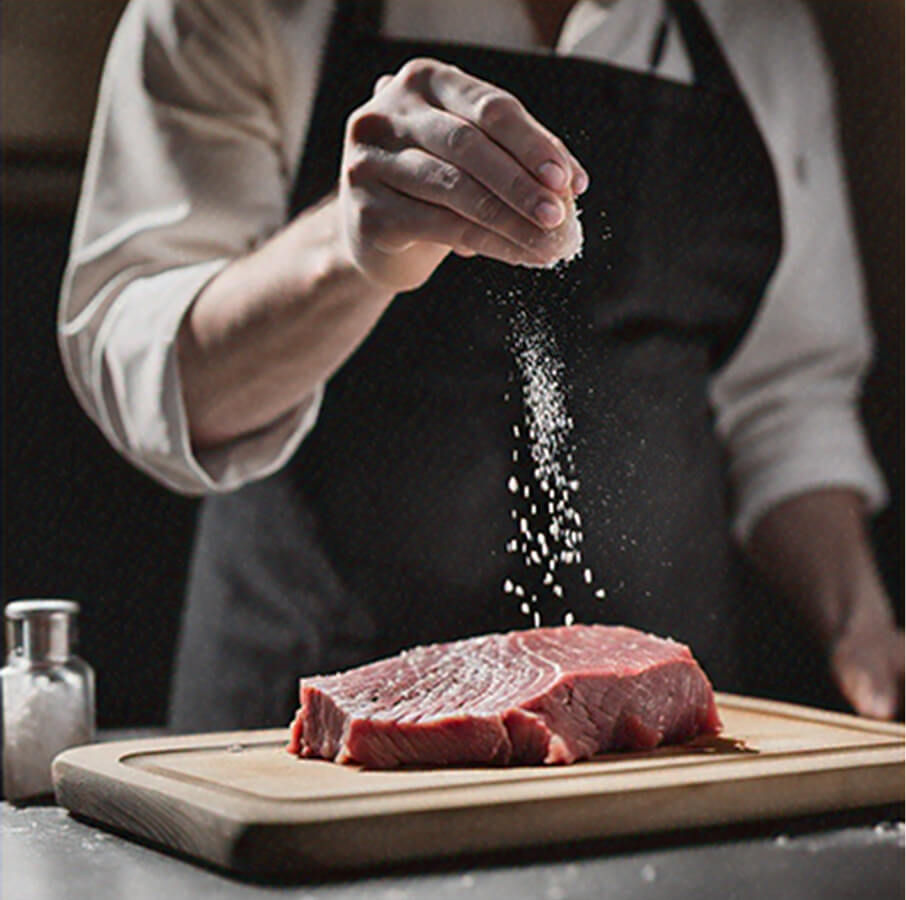


1
Season the good fresh beef fillets with salt and black pepper. Heat olive oil in a pan over high heat and sear the fillets for 2 minutes per side until it fully browned. Remove the beef from the pan and brush with a thin layer of mustard. Let it cool.
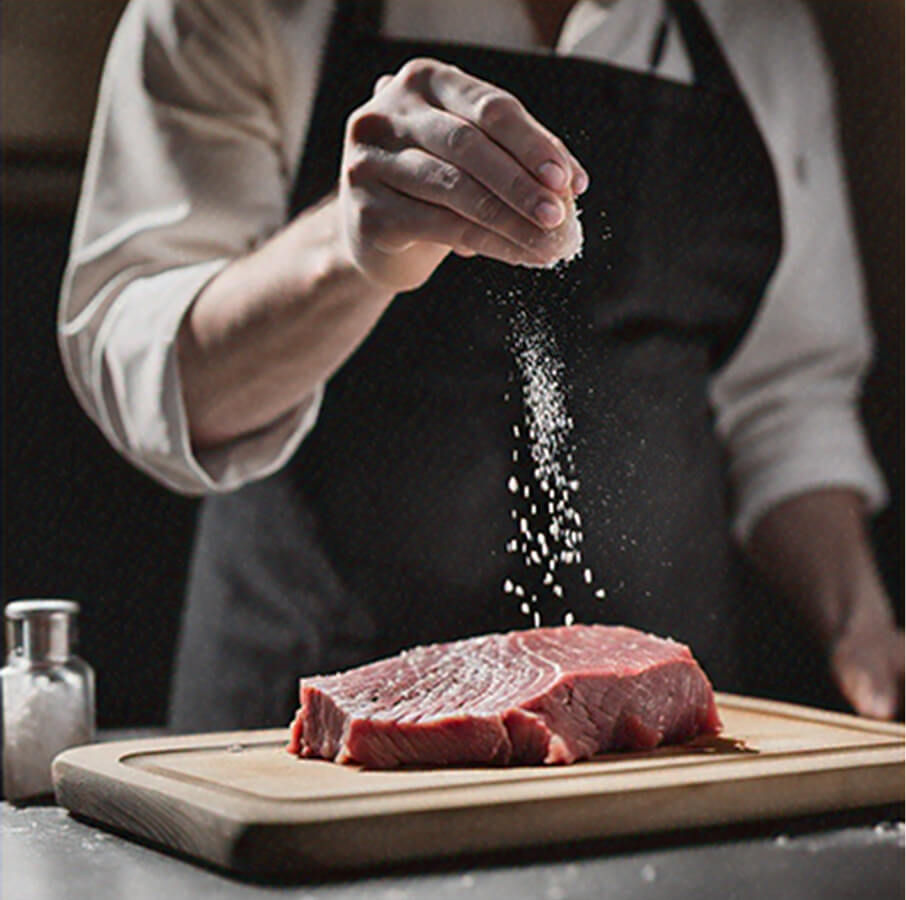


1
Season the good fresh beef fillets with salt and black pepper. Heat olive oil in a pan over high heat and sear the fillets for 2 minutes per side until it fully browned. Remove the beef from the pan and brush with a thin layer of mustard. Let it cool.
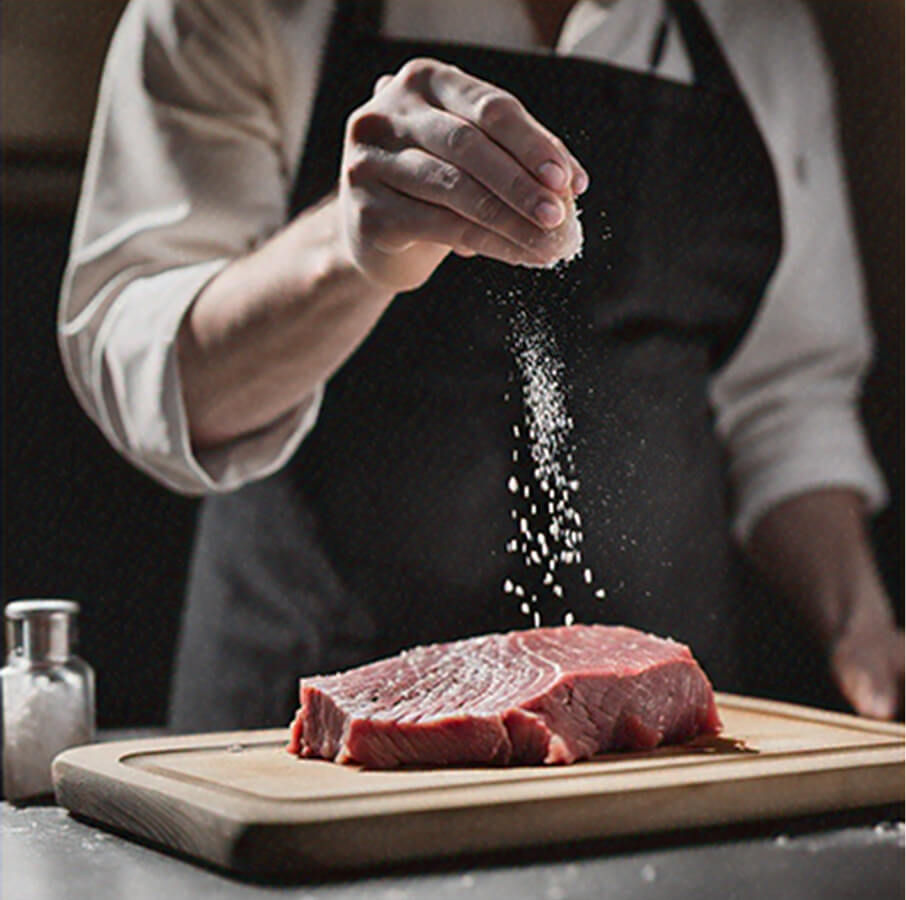


1
Season the good fresh beef fillets with salt and black pepper. Heat olive oil in a pan over high heat and sear the fillets for 2 minutes per side until it fully browned. Remove the beef from the pan and brush with a thin layer of mustard. Let it cool.
Instructions
Quality Fresh 2 beef fillets ( approximately 14 ounces each )
Quality Fresh 2 beef fillets ( approximately 14 ounces each )
Quality Fresh 2 beef fillets ( approximately 14 ounces each )
Beef Wellington
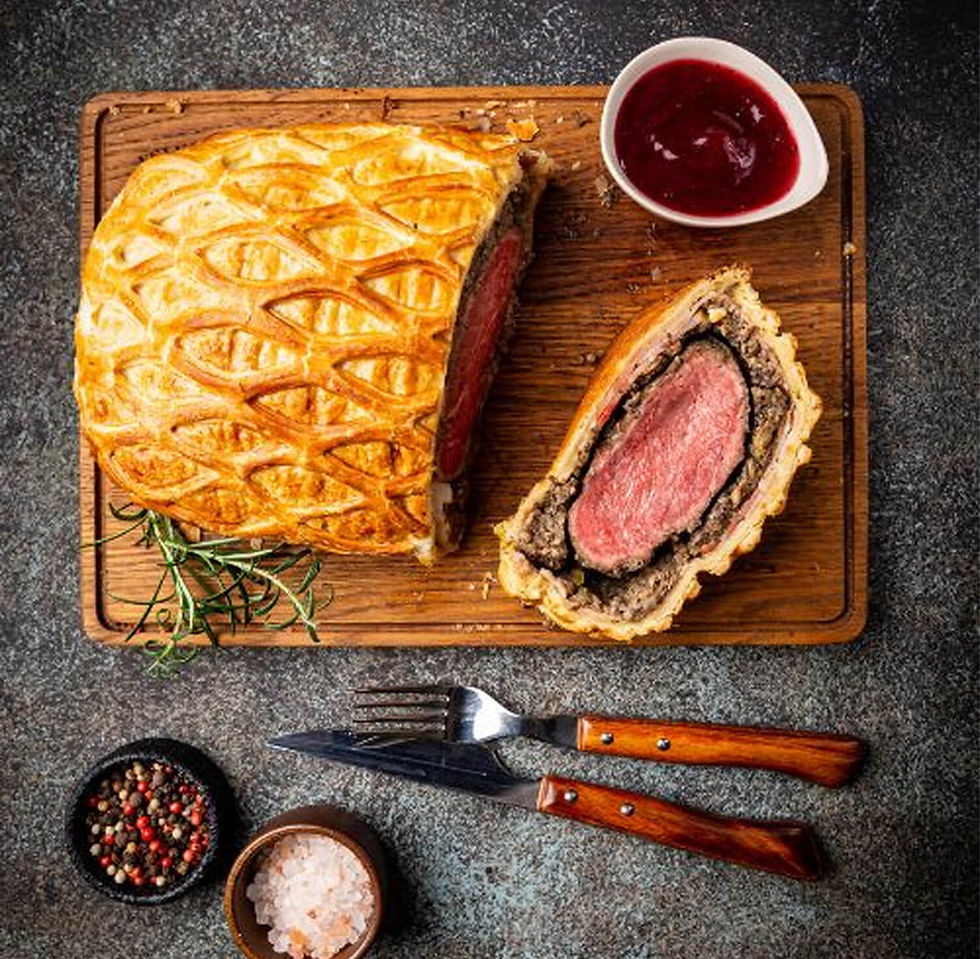
Beef Wellington
Fusion Wizard - Rooftop Eatery in Tokyo
Author Name

Beef Wellington is a luxurious dish featuring tender beef fillet coated with a flavorful mushroom duxelles and wrapped in a golden, flaky puff pastry. Perfect for special occasions, this recipe combines rich flavors and impressive presentation, making it the ultimate centerpiece for any celebration.
Servings :
4 Servings
Calories:
813 calories / Serve
Prep Time
30 mins
Prep Time
30 mins
Prep Time
30 mins
Prep Time
30 mins
Comments